Navigating the Digital Landscape: The Power and Perils of Online Reviews
What makes online reviews so influential, and why do they matter more than ever before? In this article, the authors - Arne De Keyser, EDHEC Associate Professor, Ivan Gordeliy, EDHEC Assistant Professor and Martin Wetzels, EDHEC Professor - discuss the background of online reviews and highlight some of the work they did on the motivations behind them, the role of negative scores and the challenges that come with fake reviews.
In today's digital age, where information flows freely and choices abound, online reviews have emerged as guiding beacons for consumers navigating the marketplace. Whether you are searching for a new restaurant, shopping for the latest gadget, or planning your dream vacation, you will most likely turn to online reviews to inform your decisions. TripAdvisor, for instance, features over 1 billion reviews on its website alone. But what makes these snippets of user-generated content so influential, and why do they matter more than ever before?
In this article, we will discuss the background of online reviews and highlight some of the work we did on the motivations behind online reviews, the role of negative scores and the challenges that come with fake reviews. The new Sigma Research Lab at EDHEC Business School will allow us to further delve into these, and other, research areas.

The Significance of Online Reviews
Online reviews wield tremendous influence over consumers due to their capacity to provide social proof and a sense of trust. In a world brimming with choices, reviews act as virtual word-of-mouth, helping consumers make informed decisions. When potential buyers encounter positive reviews, they perceive a good or service as more reliable and of higher quality. Furthermore, reviews often convey the experiences and satisfaction levels of previous customers, helping consumers envision their own potential interactions with a business. On the flip side, negative reviews can trigger caution or even deter consumers from making a purchase. They raise red flags, highlighting potential issues and dissuading customers from engaging with a particular brand or product.
For businesses, online reviews can be a double-edged sword. Positive reviews can bolster a company's reputation, attracting new customers and fostering loyalty among existing ones. They serve as free endorsements, building trust and credibility in the eyes of potential clients. On the contrary, negative reviews can harm a business’ image, erode trust and drive potential customers away – ultimately negatively impacting sales and profits.
Why and How Do Consumer Write Reviews?
The recent Local Consumer Review Survey 2023 reveals an impressive 98% of people read online reviews for local businesses underlining the impact of online consumer reviews for the bottom-line. Online reviews are typically rated on 5-star rating scale. In a 2013 article in Journal of Marketing (co-authored by EDHEC Professor Martin Wetzels) (1) the potential impact of negative and positive sentiments and linguistic style match on bottom-line conversion rates (instead of focusing on star ratings or rankings as opposed to prior research) for a dynamic panel of book reviews was investigated. Findings show a tapering off effect for the positive sentiment, but not for negative sentiment on conversion rates over time.This means that negative sentiment changes are showing a stronger (negative) impact on conversion rates than positive sentiment changes underlining the potential harmful effect of negative reviews on conversion rates.
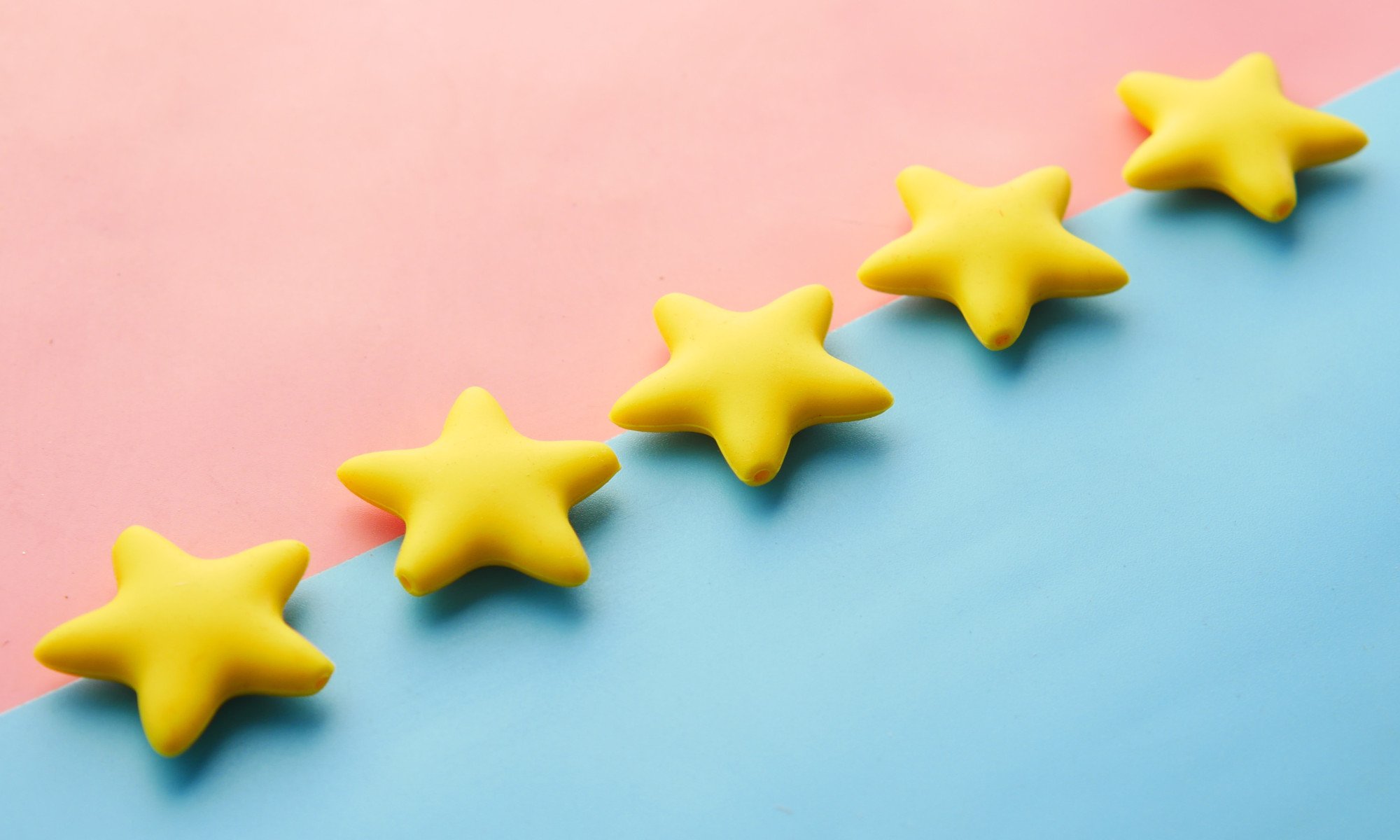
The results of the empirical study also highlight the importance of the congruence of linguistic style with different book genres suggesting that linguistic style (match) directly and conjointly with positive sentiment grow conversion rates. To improve conversion rates online retailers should consider providing guidance for writing in more congruent linguistic style for the product category and changing the order in which reviews appear, prioritizing the more congruent, positive reviews.
In a 2017 and a 2019 Journal of Consumer Research article (co-authored by EDHEC Professor Martin Wetzels) (2) (3) this research was extended using insights from linguistics using Natural Language Processing (NLP).
The 2017 follow-up research mainly explored the impact of implicit sentiment expressions (beyond individual sentiment words) and sentiment intensity (some sentiments are stronger than others) using data obtained from Amazon, Twitter (now known as “X”) and Facebook. The use of NLP allows for a finer grained analysis of implicit sentiment expressions (directive, commissive and assertive expressions) beyond the use of individual positive and negative sentiment words based on dictionaries.
Finally, in the 2019 follow-up study the potential impact of images on message sharing was explored. The findings of the study show that the use of images overall leads to more sharing of brand messages. However, the use of action-oriented (or directive) images leads to less sharing of brand messages.
The Impact of Low Review Scores
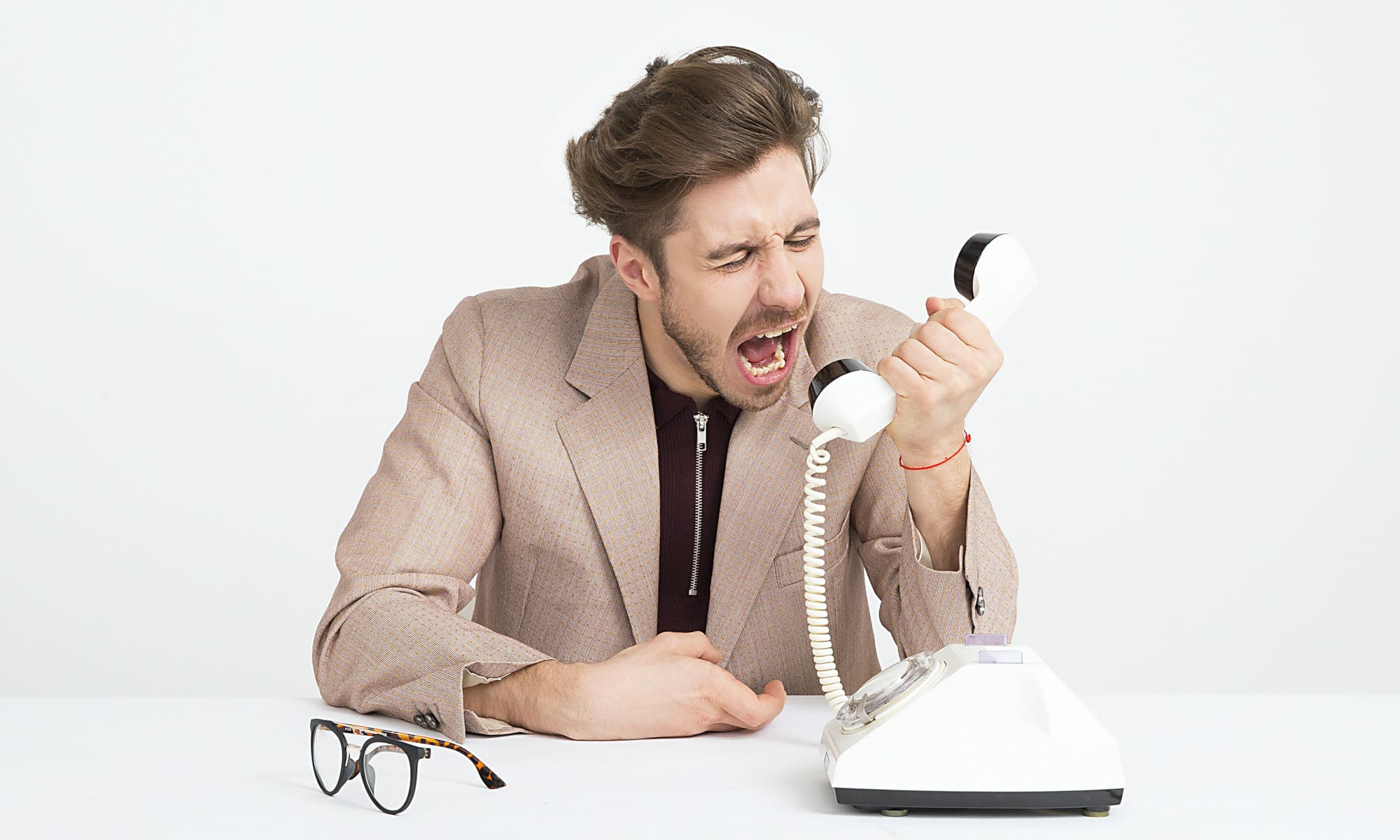
Most online reviews and scores typically lean towards the positive end of the spectrum. Amidst this sea of positivity, negative scores stand out, capturing our attention and often wielding a significant impact. Imagine receiving a negative score on a second-hand sales platform after previously enjoying positive experiences and receiving good ratings from other users. Such an experience is likely to leave you questioning what went wrong, potentially deterring further engagement with the platform.
A recent Journal of Marketing Research article (co-authored by EDHEC Associate Professor Arne De Keyser) (4) highlights how the format in which such negative scores are displayed can significantly influence consumer outcomes. When a typically high-performing entity – that is a good, a service, a customer, an employee, … – receives a negative score, presenting it in an averaged format (showing review scores as an overall average across – e.g., “Your overall average score is 4,2/5)) tends to soften the perceived negativity, in contrast to an incremental format (displaying every individual review – e.g., scores of 5/5, 5/5, 5/5, 5/5, and 1/1).
For instance, picture a situation where a ride-sharing driver, such as those on platforms like Uber or Lyft, unexpectedly receives a low rating, despite maintaining a track record of excellence. When this negative rating is presented in an averaged format, it lessens the impact, making it less detrimental to the driver's overall reputation. This, in turn, can lead to better customer retention, as passengers are more likely to consider giving the driver another chance. Conversely, when the platform intends to discourage the driver from continuing, displaying low review scores incrementally is recommended. The choice of format can either highlight or soften deviations in ratings, making it a strategic tool for companies to retain consumers, employees, and other relevant parties.
Dealing with Fake Reviews
Growing concern among scholars and regulators highlights the extensive prevalence of fake reviews on online platforms and their impact on consumer behavior. It's estimated that up to 70% of reviews in some product categories are deceptive, resulting in a potential loss of consumer welfare amounting to 12 cents for every dollar spent, as customers are misled towards inferior products.
The susceptibility of consumers to fake reviews can largely be attributed to 'truth bias' — a natural propensity to trust written statements, coupled with a lack of awareness about this inclination. Moreover, the difficulty in distinguishing truth and deception in written form exacerbates the issue. Participants in a study by a team of researchers, including EDHEC Assistant Professor Ivan Gordeliy, did not improve at detecting fake reviews, even when armed with linguistic cues to deception (5).

From a business and research standpoint, even defining what a "fake" review entails may not be straightforward. Is it inaccurate information, a breach of platform terms, or a non-user's review?
A recent study (2023) published in the Journal of Consumer Research (co-authored by Prof. Ivan Gordeliy) (6), reveals a groundbreaking approach to examining fake reviews by delving into the cognitive processes involved in their creation. Genuine reviews typically draw from episodic memory, detailing the specifics of an event—what happened, where it took place, and when. Fabricated reviews lack this dimension, exhibiting unique linguistic patterns as a result. Researchers identified five key linguistic indicators that can help distinguish real from fake: language specificity, usage of rare words, informativeness, reliance on alternative textual cues, and the presence of the three Ws (what, where, and when) of episodic memory.
Imagine describing a recent visit to the Musée d'Orsay, with fresh details such as the inefficient visitor flow or the overwhelming crowd, in contrast to a description based on pre-existing knowledge alone. These genuine reflections, steeped in recent experience, contrast with those based solely on semantic memory, offering a way to distinguish between authentic and fictitious reviews.
In response to the escalating issue of fake reviews, the research team, including Professor Gordeliy, is developing practical solutions. Their current collaboration with a major online platform seeks to apply their theoretical findings to strengthen review verification processes. This venture is a compelling call to action for businesses to invest in refining their review systems, using state-of-the-art research to stem the tide of fake reviews and uphold consumer trust.
Moving Forward – New Data Streams in the Review Realm
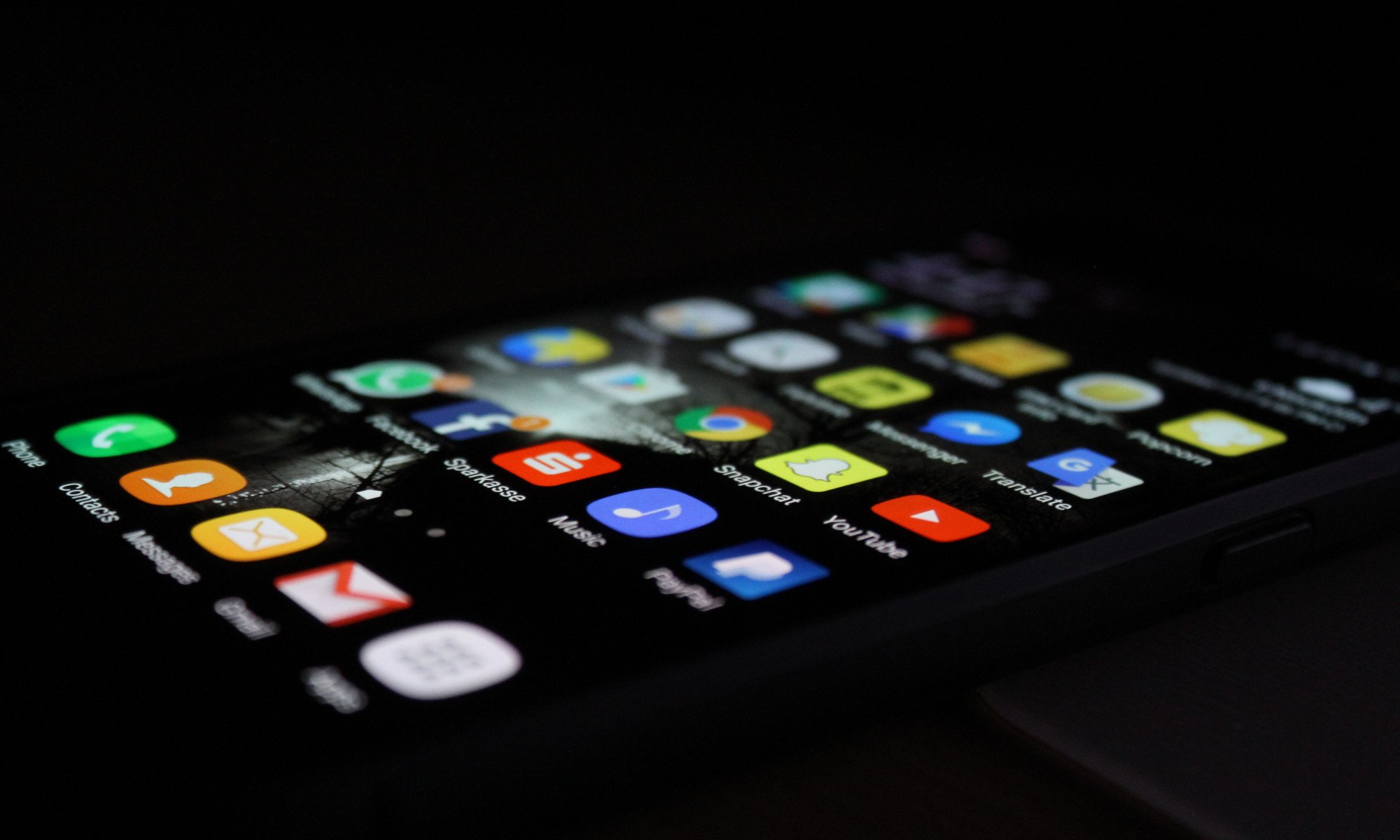
As the landscape of online reviews evolves, scholars and industry professionals must recognize the shifting modalities through which consumers share their experiences. While a vast majority of past research has honed in on written reviews, the popularity of visual and auditory mediums, such as Instagram, YouTube and TikTok, suggests that these avenues are ripe for exploration. Today, we increasingly see multimedia reviews that offer a richer, more immersive perspective on goods or services, capturing nuances that may be lost in text. For this, analytical methods like voice, image and video mining are necessary extensions of the marketer’s toolbox.
For instance, in a 2023 Journal of the Academy of Marketing Science article (co-authored by EDHEC Professor Martin Wetzels) (7) the impact of artificiality (AI) and intelligence on digital voice assistant evaluation is explored through such new methods. As the digital age continues to redefine the way we share and consume reviews, it is imperative for marketing to broaden its horizons, leveraging advanced technologies and methodologies to truly understand the multifaceted nature of consumer feedback.
References
(1) Ludwig, S., Ruyter, J.C. de, Brüggen, E.C., Pfann, G. and Wetzels, M.G.M. (2013), More than Words: The Influence of Affective Content and Linguistic Style Matches in Online Reviews on Conversion Rates, Journal of Marketing, 77 (1), 87-103. https://doi.org/10.1509/jm.11.0560
(2) Villarroel-Ordenes, F., Ludwig, S., Ruyter, K. de, Grewal, D. and Wetzels, M. (2017), Unveiling What Is Written in the Stars: Analyzing Explicit, Implicit and Discourse Patterns of Sentiment in Social Media, Journal of Consumer Research, 43 (6), 875-894. https://doi.org/10.1093/jcr/ucw070
(3) Villarroel-Ordenes, F., Mahr, D., De Ruyter, K., Wetzels, M., Ludwig, S., and Grewal, D. (2019), Cutting through Content Clutter: How Speech and Image Acts Drive Consumer Sharing of Social Media Brand Messages, Journal of Consumer Research, 45 (5), 988-1012. https://doi.org/10.1093/jcr/ucy032
(4) Lembregts, C., Schepers, J. & De Keyser, A. (2024). Is it as bad as it looks? Judgements of quantitative scores depend on their presentation format. Journal of Marketing Research (forthcoming). https://doi.org/10.1177/00222437231193343
(5) Kronrod, A., Lee, J. K., & Gordeliy, I. (2017). Detecting fictitious consumer reviews: A theory-driven approach combining automated text analysis and experimental design. Marketing Science Institute Working Papers Series, 17–124.
(6) Kronrod, A., Gordeliy, I., & Lee, J. K. (2023). Been There, Done That: How Episodic and Semantic Memory Affects the Language of Authentic and Fictitious Reviews. Journal of Consumer Research, Vol. 50, Issue 2, August 2023, Pages 405–425. https://doi.org/10.1093/jcr/ucac056
(7) Schweiger, E., Breßgott, T., Guha, A., Grewal, D., Wetzels, M. & Mahr, D. (2023), How artificiality and intelligence affect voice assistant evaluations, Journal of the Academy of Marketing Science, 51(4), 843-866. https://doi.org/10.1007/s11747-022-00874-7
Other Relevant Background Literature
- Babić Rosario, A., de Valck, K. & Sotgiu, F. (2020). Conceptualizing the electronic word-of-mouth process: What we know and need to know about eWOM creation, exposure, and evaluation. Journal of the Academy of Marketing Science, 48, 422-448.
- Köcher, S. & Köcher, S. (2021). The mode heuristic in service consumers’ interpretations of online rating distributions. Journal of Service Research, 24(4), 582-600.
- Mousavi, J., Singh, S. N., Chatterjee, P. & Tamara Masters (2024). Unveiling stars: How graphical displays of online consumer ratings affect consumer perception and judgement. Journal of Marketing Research, forthcoming.
- Tirunillai, S. & Tellis, G. J. (2012). Does chatter really matter? Dynamics of user-generated content and stock performance. Marketing Science, 31(2), 198-215.
- TripAdvisor (2022). Travelers Push Tripadvisor Past 1 Billion Reviews & Opinions!
Pictures credits (from top to bottom)
Photo de Volodymyr Hryshchenko sur Unsplash - Photo de Shayna Douglas sur Unsplash - Photo de Towfiqu barbhuiya sur Unsplash - Photo de Icons8 Team sur Unsplash - Photo de Victor He sur Unsplash - Photo de Rami Al-zayat sur Unsplash